In recent years, advancements in artificial intelligence have revolutionized life sciences, enabling unprecedented breakthroughs in understanding biomolecules and their interactions. Despite these strides, the field of antibody modeling and design remains fraught with challenges, requiring innovative solutions to accelerate drug discovery and development.
BioGeometry, a leader in generative AI for protein design, is dedicated to overcoming these hurdles. Today, we are excited to announce our latest innovation, GeoFlow, a next-generation AI model designed to transform the landscape of antibody design.
GeoFlow leverages state-of-the-art geometric deep learning and flow matching technologies[1] to excel in both antigen-antibody complex structure prediction and de novo antibody design. The model not only matches the performance of AlphaFold 3 (the latest model developed by Google DeepMind and Isomorphic) in the task of antibody-antigen docking, but also offers unique antibody design capabilities that push the boundaries of what generative AI can achieve in the life sciences.
To empower researchers and scientists, BioGeometry is making GeoFlow available for non-commercial testing. We invite the scientific community to explore its capabilities and join us in pioneering the next wave of advancements in antibody design. Visit geobiologics-lite.biogeom.com to get started.
Generative AI: Pioneering the Future of Macromolecular Design
Traditional discriminative AI can be compared to a music critic, who listens to and evaluates songs. Generative AI, on the other hand, is akin to a singer/producer, who possesses the ability to create new songs.
The leap from data labeling to data generation has greatly expanded AI’s application scope. For instance, in structure prediction, generative AI can quickly sample numerous conformations; in protein design, it efficiently explores protein space, crafting complex molecules with desired functions.
As a pioneer in generative AI for macromolecules, BioGeometry has deep expertise in diffusion models (modeling noise-to-distribution processes) and flow matching (modeling distribution-to-distribution processes).
Diffusion models has enjoyed great success in image generation and more recently video generation, with Midjourney and Sora being prominent examples. BioGeometry’s team applied diffusion models to 3D molecular structure generation as early as 2021 [2,3], being among the first to do so. GeoDiff, a key paper in the field, was one of the top 50 most cited AI papers in 2022 [4].
Building on this technical foundation, BioGeometry developed GeoFlow, a generative AI model for antibody modeling and design. GeoFlow’s architecture leverages geometric deep learning and the latest flow matching model, excelling in both antigen-antibody complex structure prediction and antibody design. GeoFlow employs geometric deep neural networks (rather than Transformers) as the foundational architecture to better model the atomic relationships in 3D space. The flow matching model enhances efficiency and robustness in training and inference compared to diffusion models.
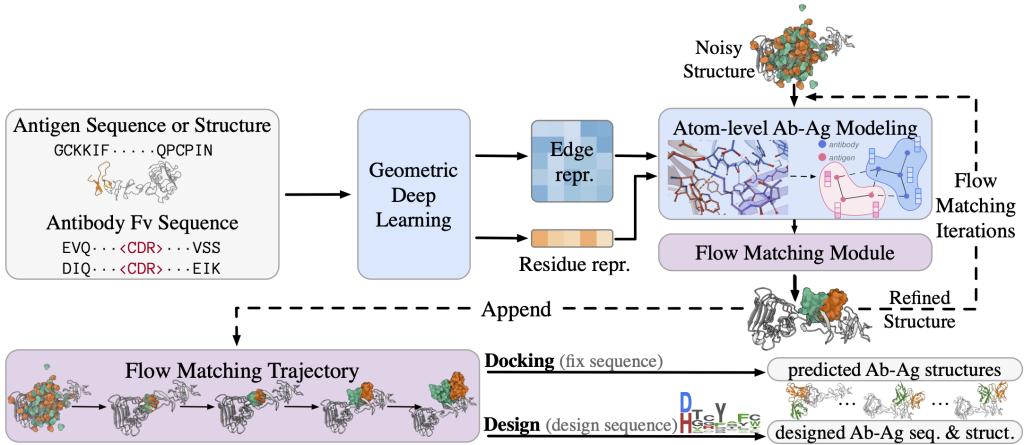
Matching AlphaFold 3 in Antigen-Antibody Docking
We evaluated GeoFlow’s performance in predicting antigen-antibody complex structures, a critical task in antibody drug discovery. Previous methods, including traditional methods (like HDock and ZDock) and deep learning models (like AlphaFold 2 Multimer), have yet to produce satisfactory results in this task.
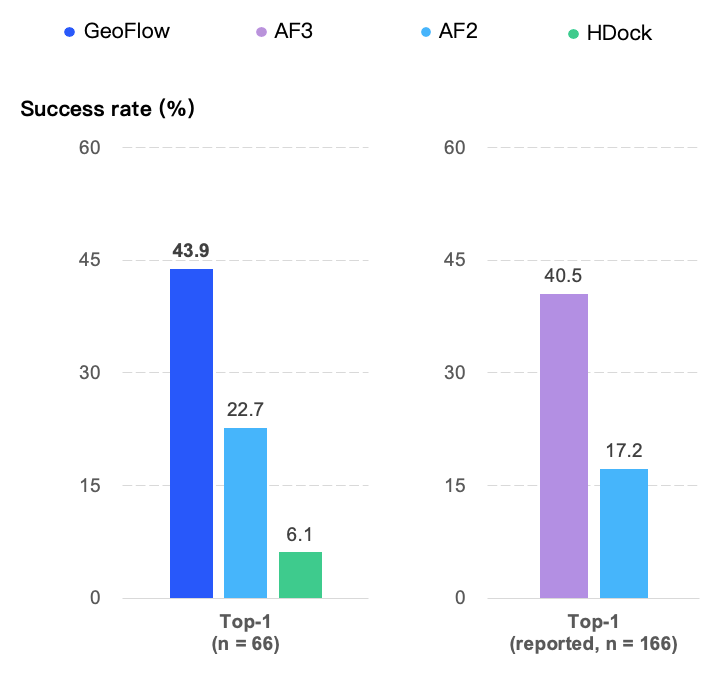
In a test set of 66 antigen-antibody complexes (released after 2023), GeoFlow’s Top-1 success rate (DockQ level “Acceptable” or above) reached 43.9%, matching AF3’s reported performance and nearly twice the performance of AF2 Multimer. HDock, though able to generate a diverse pool of predicted structures, have low scoring accuracy and thus limited practical value.
De Novo Antibody Design and Validation
Unlike AF3, GeoFlow goes beyond structure prediction tasks and can also perform de novo antibody design and optimization. Traditional discriminative AI struggles with macromolecule design because it can only repeatedly score low-quality samples, trying to find the good ones, akin to finding a needle in a haystack.
Generative AI revolutionizes this process by directly generating high-quality samples. Given an antigen structure and specific epitope, GeoFlow generates new antibody molecules.
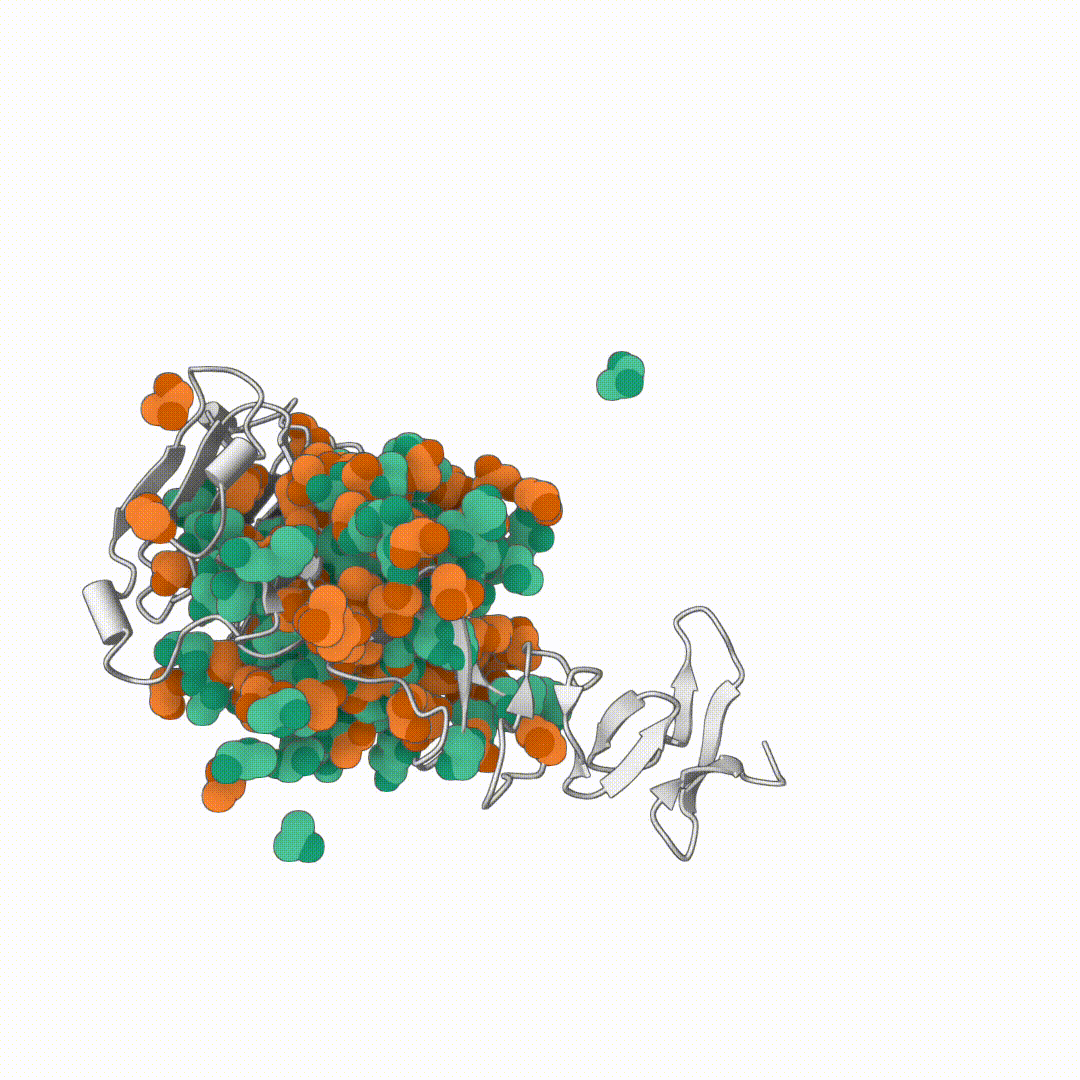
For the HER2 target, using Herceptin’s binding epitope, we generated a small antibody library with GeoFlow and screened it using a phage display library. Among the ten selected candidates:
- Binding activity: 6 molecules showed ELISA binding comparable to Herceptin, reaching nanomolar levels. BLI results indicated that molecules 1 and 3 had 2-3 times higher affinity than Herceptin.
- Binding epitope: Competitive ELISA revealed strong competition with Herceptin for these 6 molecules, suggesting similar binding epitopes.
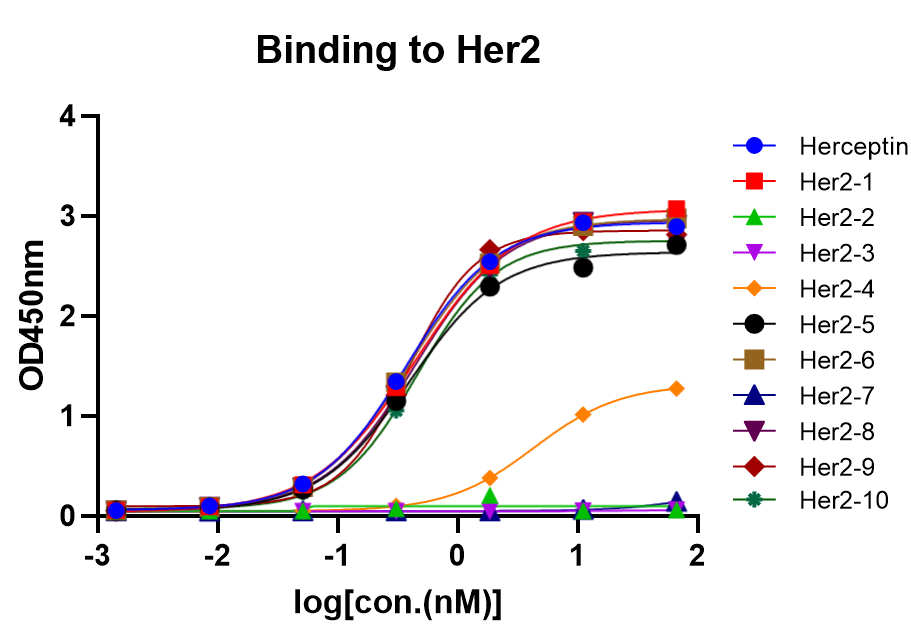
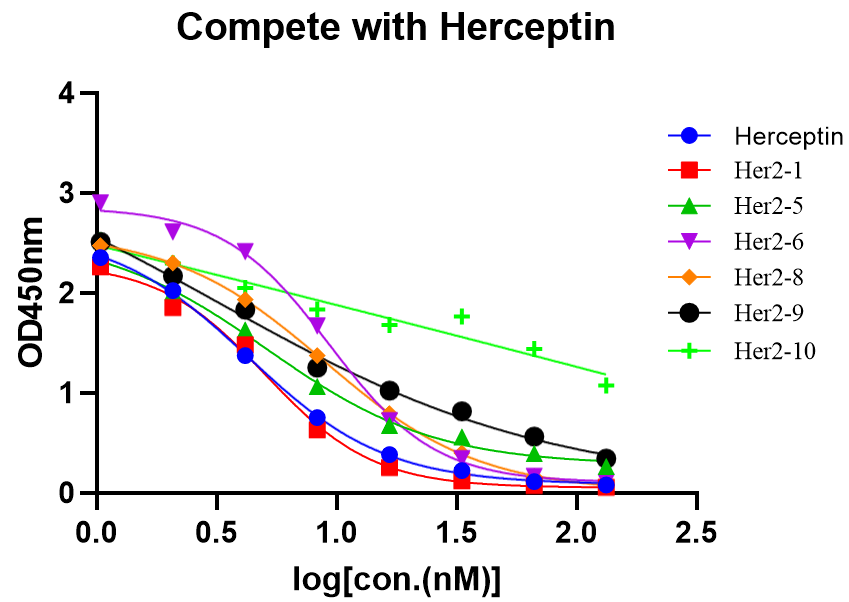
These results highlight generative AI’s vast potential in de novo macromolecule design. As a digital biology pioneer, BioGeometry is actively advancing towards larger design regions, more challenging targets, and higher affinities.
BioGeometry Opens GeoFlow for Non-Commercial Use
BioGeometry’s generative AI solutions span all stages of macromolecular design, screening, and modification. Through the one-stop antibody discovery platform GeoBiologics, we have delivered tangible benefits to our clients and partners.
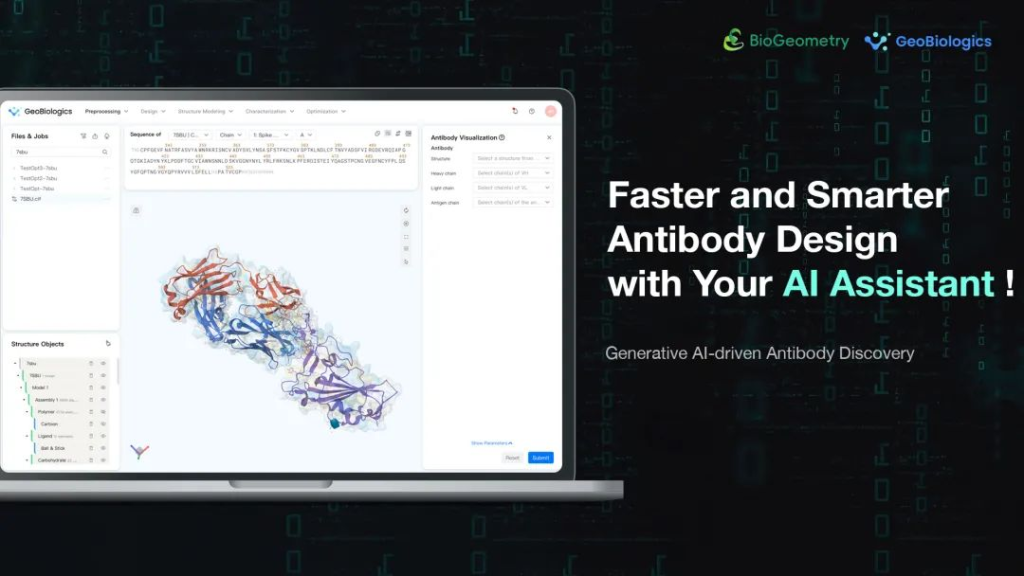
To give more users access to powerful generative AI tools, we are opening the GeoFlow model for non-commercial use. You may visit geobiologics-lite.biogeom.com and start exploring now.
Looking forward, BioGeometry will continue developing cutting-edge generative AI algorithms and applying them to the biopharmaceutical and synthetic biology industries. Our vision is to build a greener, smarter, and healthier world, one protein at a time.
Related Articles
[1] Lipman, Yaron, et al. “Flow matching for generative modeling.” 11th International Conference on Learning Representations (ICLR 2023)
[2] Shi C, Luo S, et al. “Learning gradient fields for molecular conformation generation.” 38th International Conference on Machine Learning (ICML 2021)
[3] Xu M, et al. “Geodiff: A geometric diffusion model for molecular conformation generation.” 10th International Conference on Learning Representations (ICLR 2022)
[4] https://www.zeta-alpha.com/post/must-read-the-100-most-cited-ai-papers-in-2022
[5] Abramson, Josh, et al. “Accurate structure prediction of biomolecular interactions with AlphaFold 3.” Nature (2024): 1-3.